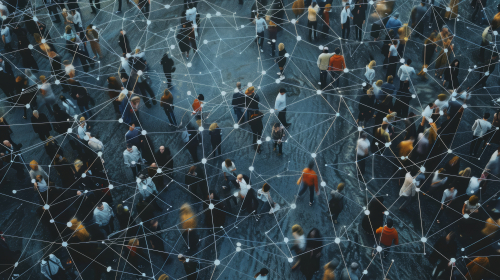
By Julia Schwarz
As artificial intelligence increases its role in everything from education testing to medical diagnoses, ensuring the systems’ fairness is a key goal for researchers and policymakers. Currently, AI engineers evaluate fairness with a single leaderboard number, but research from Princeton Engineering shows that reducing fairness to a single metric could lead to societal harm.
“Things like fairness and even intelligence are multidimensional,” said researcher Angelina Wang, a former graduate student at Princeton who has been appointed to the faculty at Cornell Tech in New York City.
In an article in the journal Patterns, Wang and Olga Russakovsky, associate professor of computer science and associate director of the Princeton AI Lab, argue for a multidimensional approach in which fairness is evaluated on several levels depending on the context of the application.
For example, consider two large language models being used to select scholarship recipients. One LLM might take a race-blind approach, the other a race-aware approach. Depending on the context — the rules of the scholarship and what it is selecting for — one LLM might be better than the other. Or consider an image captioning algorithm that infers the gender of individuals in the images. Gender inference may be the preference of low-vision or blind users, but not transgender or non-binary individuals. A suite of tests that reveals how gender or race is treated broadly will help users understand which system may work best for them.
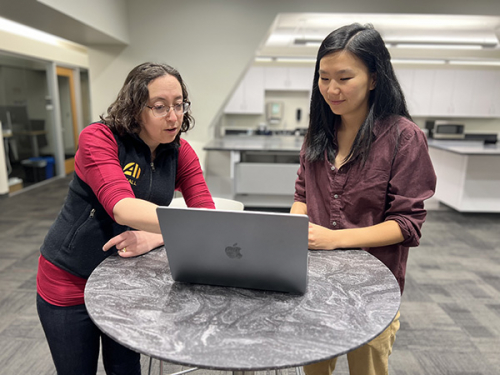
“Depending on the context, one model may be more fair than another, and a benchmark suite of disaggregated metrics can tell you that,” said Wang.
According to Russakovsky, an expert in computer vision, computer scientists have primarily been focused on building more powerful AI algorithms. “We’ve seen a shift in the past 10 or 15 years,” she said, “to a greater focus on the data and on downstream applications, and this has raised many questions about fairness.” The first step to answering these questions, she said, is to move away from measuring fairness with a single metric and instead adopt a more multidimensional, comprehensive approach.
Understanding exactly how many benchmark tests are required for this, how the data should be presented and who should evaluate it is the work of ongoing research for Wang and Russakovsky.
Being able to understand how AI models work and whether they are fair is incredibly important, said Russakovsky. “We have a long history of building technology that leaves populations behind,” she said. “Like building seat belts that don’t work for women, because we never tested them on women. Like creating a test for kidney function that makes it harder for African Americans to get treatment.”
As we enter the age of AI, she said, thinking about how new tools impact fairness and affect different groups of people is paramount. “If we don’t, we will join that long line of tech solutions that are deeply problematic.”
The paper, “Benchmark suites instead of leaderboards for evaluating AI fairness,” was published Nov. 8 in Patterns. In addition to Russakovsky and Wang, coauthors include Aaron Hertzmann of Adobe Research.