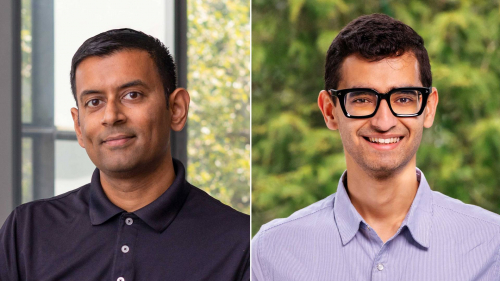
By Liz Fuller-Wright, Princeton Office of Communications
In the two years since ChatGPT brought artificial intelligence out of science fiction and into the public conversation, AI has been both wildly hyped and harshly vilified.
The acclaimed new book “AI Snake Oil” by Princeton AI scholars Arvind Narayanan and Sayash Kapoor offers readers a smart new perspective, with surprising insights on what should excite people most, and what should alarm us.
Narayanan is a professor of computer science and the director of Princeton’s Center for Information Technology Policy (CITP). Kapoor is a former Facebook engineer now completing a Ph.D. in computer science at Princeton.
“AI Snake Oil: What Artificial Intelligence Can Do, What It Can’t, and How to Tell the Difference,” has made Top 10 and year-end recommendation lists from Publishers Weekly, Nature, Bloomberg, USA Today and others. It is published by Princeton University Press.
We asked Narayanan and Kapoor to distill some of the book’s key messages and tell us more about their work at Princeton. The interview has been edited and condensed.
What is ‘AI Snake Oil’ — both the concept and the book?
Narayanan: AI snake oil, as a concept, is AI that doesn’t work as advertised and probably can’t work as advertised. The “AI Snake Oil” book is about the foundational knowledge that people will need to separate the hype from what is real.
AI is a very polarizing topic. Some people promise that it’s going to revolutionize our lives and the economy. Others are skeptical and think it’s all a bunch of hype. We wrote this book because there is something to both of these narratives.
Some aspects of AI have genuinely made remarkable technical progress. But on the other hand, there is so much flawed AI that’s being sold.
People are very confused, and we think they need to have some level of understanding of this technology so they can make informed decisions.
Kapoor: We have seen a number of examples where companies will sell products that literally cannot work, like the hiring tools that claim they can predict how well a candidate would do at a job, based on a 30-second video. These are tools not backed by any scientific evidence.
The book is about distinguishing between applications of AI that work and those that don’t. It is meant to empower readers to be able to make these decisions in their day-to-day lives.
Arvind, you’ve said that when it comes to AI, people are afraid of the wrong things.
Narayanan: In my years of studying the risks of technology, there’s one aphorism that’s been very helpful for me, from cybersecurity expert Bruce Schneier. He says if it’s in the news, don’t worry about it.
The kinds of risks that we see in the news are exotic. But the kinds of harms that are already widespread — that are happening to people every day — those you don’t hear about.
A big part of my research agenda has been driven by the importance of rigorously analyzing what the actual risks and harms are. When it comes to AI, one important way in which I’ve tried to do that is to distinguish between generative AI and predictive AI.
Generative AI, like ChatGPT, is in the news for understandable reasons, and it’s what policymakers are primarily concerned with these days.
But when you look at the kind of AI that is actually widely deployed — that will be invisibly making massively consequential decisions about people’s lives — that’s predictive AI.
These are algorithms that are used to make life-altering decisions about people by entities in positions of power — governments or companies — and the way these algorithms work is by making predictions about people’s future behavior or their outcomes.
What kinds of decisions is predictive AI making?
Kapoor: It might be used to decide who gets a job. It might be used to decide who is released on bail, what the bail amount is. The stakes couldn’t be any higher.
Narayanan: In the medical system, AI is used to predict, “This person needs 17 days in the hospital to recover.” It’s just an oddly precise number, and there are horror stories of people whose insurance coverage is denied after that number of days has elapsed, even though they still need care.
In hiring, if AI doesn’t like your application, you might get rejected not just from one job, but over and over again, because they’re all using the same AI vendor.
It’s hard to predict the future, and predictive AI doesn’t change that. It doesn’t mean we should never use it, but we should be skeptical and cautious about using it.
In your book, you say using “AI” to refer to both generative and predictive AI — and other loosely related technologies, like image recognition — is as problematic as using “vehicle” without differentiating between bikes, cars and spaceships.
Kapoor: In everything that we call AI, the underlying technologies often have nothing to do with each other.
We have made huge advances in generative AI over the last decade. This is a technology that has literally been improving year over year at frankly a very impressive rate. On the other hand, predictive AI is based on decades-old tools.
When you talk about predictive AI and generative AI in the same way, it leads to public confusion. We’ve seen this with researchers who talk about AI, with companies selling AI and in news articles that are talking about what are essentially glorified Excel spreadsheets — with images of the Terminator.
Narayanan: Something like ChatGPT, which is generative AI, has very little in common with [the predictive] AI that banks might use to calculate someone’s credit score.
These are two very, very different technologies, and we have to talk about them differently.
Generative AI is what people worry about a lot, whether it’s political disinformation or “Will AI become self-aware and end humanity?” But when you look at what kind of AI is really having the most impact on people’s lives? By far, predictive AI.
Both of you chose to leave the Silicon Valley area to come to Princeton.
Kapoor: Before I started my Ph.D. here, I worked on machine learning at Facebook. And while it was a perfectly fine job, I really wanted to think more deeply about how tech impacts society.
When you’re working in Silicon Valley, it’s very easy to be consumed by the next six months or the next quarter, rather than how technology will affect society over the next 10 years. I wanted to be able to think long-term.
Narayanan: One thing I really enjoy is my ability to collaborate with experts in other disciplines, like sociologist Matt Salganik. Some of the work that I value most has come through those collaborations.
And, frankly, the fact that we are at a little bit of a distance from Silicon Valley, I’ve found to be very advantageous for a lot of the kinds of work that I do, because I can define my agenda without following the imperatives of the tech industry. In many ways, I can challenge the priorities that the industry has set.
Princeton’s Center for Information Technology Policy (CITP) is uncommon and possibly unique in that it has equal strengths on the technical side of AI and on the policy side. How has its focus on interdisciplinary collaborations affected your work?
Narayanan: Often, what we regulate in tech policy is not the technology itself, but human behavior around it, so it’s vitally important to understand the sociological aspects of it and the legal aspects of it. It goes without saying that we need humanistic expertise as well, and CITP is a place where all those kinds of expertise can mix.
One of the nice things about Princeton in general and CITP in particular is freedom. Our scholars set their own agendas.
Kapoor: I’ve worked in CITP for the last four years or so, and in that time, my office neighbors have been a sociologist, a lawyer, a computer scientist and a journalist. These are top people from their fields who can guide us as we make our way through this very important space of AI policy and AI research.
In terms of AI, what are you most worried about in the coming years?
Kapoor: One thing that is quite worrying right now is the amount of industry influence. Only the largest labs can work on some types of large language models, so you really need to rely on technology from these companies, like OpenAI and Google and Facebook, to work on the latest and greatest in AI.
That’s an issue because it means that the entire technical agenda can be driven by these companies — and we are also seeing AI companies consistently being the ones who spend the most in terms of political lobbying.
And on the flip side, what gives you hope?
Kapoor: I’m really hopeful about the integration of AI in a large number of knowledge workers’ day-to-day workflow.
We already rely on a large number of AI technologies every single day, but because they work very, very well, we let them fade into the background. Spellcheck was once thought to be an intractable problem, and now it happens automatically.
We are building toward a vision where “AI snake oil” can be easily discussed and discarded, and we can focus on the applications of AI that are truly beneficial and that can fade into the background.
Narayanan: I think generative AI is a genuinely new and interesting technology. There’s no question about that. We’re going to find new applications for it.
We only call something “AI” when it’s at the cutting edge, when its societal implications are dubious. But once it starts working really well, we just take it for granted as a part of our digital environment. Things like autocomplete and spellcheck, these are the kinds of AI we want more of. We think a lot of kinds of AI that are problematic today are one day going to transition into this category.
Take self-driving cars. I hope that within a couple of decades, most car rides will involve automation, to cut down on the roughly 1 million auto-related fatalities that we have around the world each year.